Publications
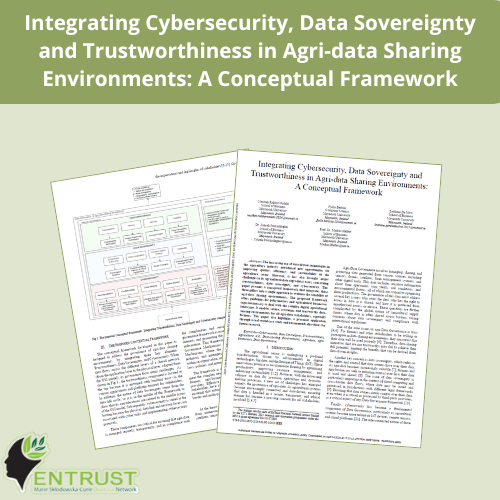
Integrating Cybersecurity, Data Sovereignty and Trustworthiness in Agri-data Sharing Environments: A Conceptual Framework
The increasing use of data-driven technologies in the agriculture industry introduced new opportunities for improving quality, efficiency, and sustainability in the agriculture sector. However, it has also brought major challenges to its agricultural data (agri-data) users, concerning trustworthiness, data sovereignty, and cybersecurity. This paper presents a conceptual framework that integrates these three pillars into a single approach to evaluate the reliability of agri-data sharing environments. The proposed framework offers guidelines for policymakers and agricultural businesses (agri-businesses) to deal with the complex digital agricultural landscape. It enables secure, sovereign, and trustworthy data- sharing environments for all agri-data stakeholders, especially farmers. This paper also highlights a practical application through a real-world case study and recommends directions for future research.
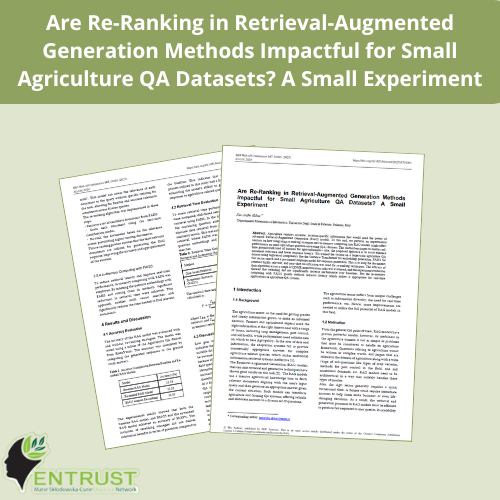
Are Re-Ranking in Retrieval-Augmented Generation Methods Impactful for Small Agriculture QA Datasets? A Small Experiment
Efficiently generating knowledge from small-scale, question-answering agricultural datasets is a complex task. This study by Nur Arifin Akbar explores how Retrieval-Augmented Generation (RAG) methods can improve knowledge extraction while addressing computational limits in agricultural informatics.
By combining Sentence Transformers, FAISS vector search, and pre-trained language models, this research displays how re-ranking strategies improve document relevance and response accuracy for specialized agricultural queries. It also analyses the computational trade-offs in retrieval performance and latency reduction.
Published in BIO Web of Conferences (ICOSIA 2024), this study offers new insights into applying advanced natural language processing techniques to specialised, small-scale agricultural information repositories.
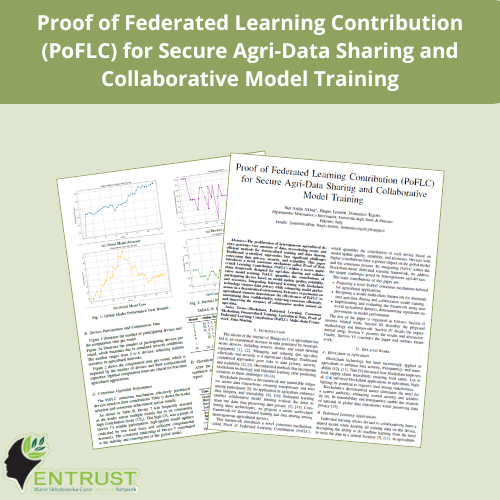
Proof of Federated Learning Contribution (PoFLC)for Secure Agri-Data Sharing and Collaborative Model Training
As farms generate more data from connected devices, securely sharing and using this data is a growing challenge. This research introduces Proof of Federated Learning Contribution (PoFLC), an advanced method that integrates federated learning principles with blockchain technology to protect privacy while improving the performance of collaborative models.
Developed by Nur Arifin Akbar, Biagio Lenzitti, and Domenico Tegolo from the University of Palermo, this novel approach quantifies device contributions through an evaluation of model update quality, reliability, and computational resources. Tested on diverse agricultural datasets, PoFLC has proven effective in maintaining data confidentiality while improving the accuracy of machine learning models. Presented at the IEEE Conference in Ishikawa, Japan (November 20-21, 2024), this research advances decentralised agricultural data science, offering a concept for secure and intelligent data collaboration.
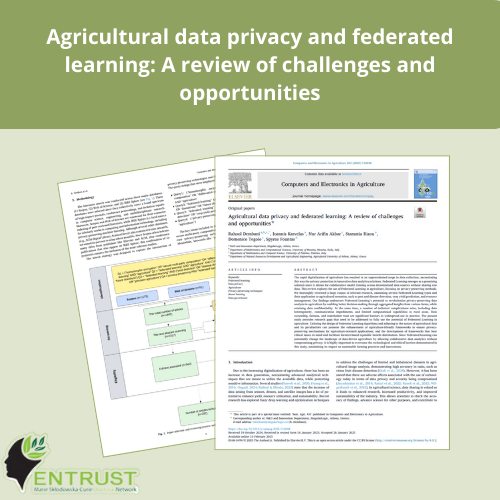
Agricultural data privacy and federated learning: A review of challenges and opportunities
Rahool Dembani, Ioannis Karvelas, Nur Arifin Akbar, Stamatia Rizou, Domenico Tegolo, Spyros Fountas
The rapid digitalization of agriculture has resulted in an unprecedented surge in data collection, necessitating privacy protection of agricultural data in innovative data analytics solutions. Federated Learning emerges as a promising solution since it allows for collaborative model training across decentralized data sources without sharing raw data. This review explores the use of Federated Learning in agriculture, focusing on privacy-preserving methods. We examined several Federated Learning types and their applications in agricultural scenarios, such as pest and disease detection, crop yield prediction, and resource management. Our findings underscore Federated Learning’s potential to revolutionize privacy-preserving data analysis in agriculture by enabling better decision-making through aggregated insights from various farms, while retaining data confidentiality.
At the same time, several technical complications arise, including data heterogeneity, communication impediments, and limited computational capabilities in rural areas. Data ownership, fairness, and stakeholder trust are significant barriers to widespread use of Federated Learning in practice. The study highlights key research gaps and suggests ways to adapt Federated Learning to agricultural needs, ensuring both privacy protection and fair benefits for farmers. Overcoming these challenges is crucial for unlocking Federated Learning’s full potential in sustainable and data-driven farming.
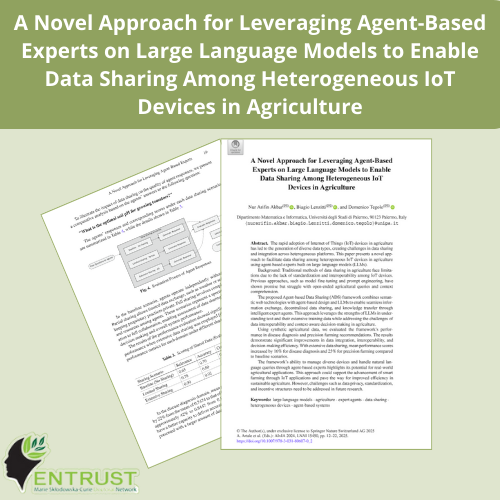
A Novel Approach for Leveraging Agent-Based Experts on Large Language Models to Enable Data Sharing Among Heterogeneous IoT Devices in Agriculture
This paper by Nur Arifin Akbar, Biagio Lenzitti, and Domenico Tegolo explores a new way to improve data sharing among different Internet of Things devices in agriculture using agent-based experts supported by large language models (LLMs). By combining autonomous “agents,” decentralized data storage, and LLMs for understanding queries, the framework aims to boost collaboration, data integration, and more informed decision-making. Tests on synthetic farming data showed clear gains in diagnosing plant diseases and providing tailored precision-farming advice. However, challenges relating to data privacy, standardization, and incentives still need to be addressed for wider real-world use.

Data value creation in agriculture: A review
Havva Uyar, Ioannis Karvelas, Stamatia Rizou, Spyros Fountas
Agricultural data is a valuable resource for driving innovation, enhancing efficiency and promoting sustainability in farming. But how is the value of this data perceived and implemented in agriculture? This review article, authored by EnTrust DC Havva Uyar, investigates key ways agricultural data generates value, such as improving processes, predicting outcomes, and tailoring solutions. The research highlights that agricultural data grows more valuable with reuse, urging a shift in focus from data ownership to the ownership of the value derived from the data. These insights highlight the need for robust frameworks and further research to realise the vast potential and value of agri-data.

Building trust: A systematic review of the drivers and barriers of agricultural data sharing
This systematic review by EnTrust DC Clare Sullivan, examines the key drivers and barriers to sharing agricultural data in smart farming. The research identifies factors like improved decision-making, collaboration, and clear data ownership as motivators, while mistrust, privacy concerns, and technical challenges act as obstacles. The findings highlight the need for better communication, trust-building, and practical solutions to encourage agricultural data sharing. These insights aim to support policies and practices that make agri-data sharing more accessible and effective.
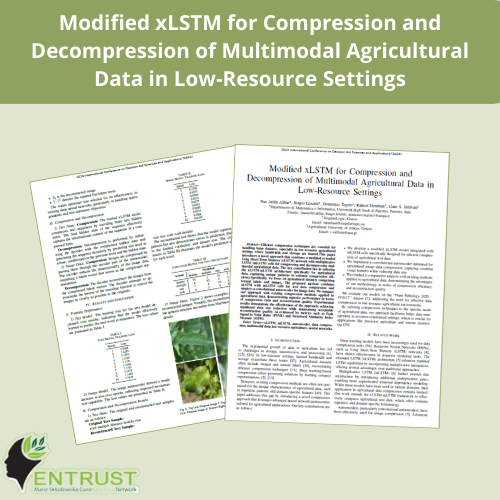
Modified xLSTM for Compression and Decompression of Multimodal Agricultural Data in Low-Resource Settings
This paper by Nur Arifin Akbar, Biagio Lenzitti, Domenico Tegolo, Rahool Dembani, and Clare S. Sullivan addresses the challenge of data management in agricultural settings with limited technological infrastructure. By developing a novel compression technique using a modified extended Long Short-Term Memory (xLSTM) network with multiplicative LSTM cells, the researchers created an innovative approach to compress text and image data from agricultural datasets. The method results in significant reductions in the size of data while maintaining acceptable reconstruction quality. This is particularly useful for precision agriculture and remote monitoring in resource-constrained environments. The approach shows promise in improving data handling efficiency, though challenges remain in preserving fine image details and expanding the text vocabulary.